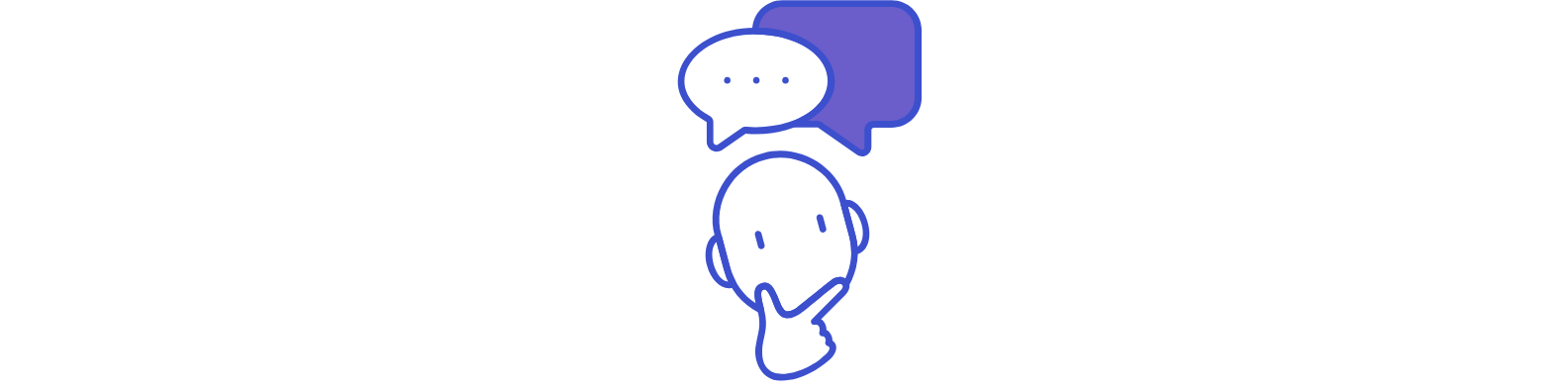
Have you ever wondered why some businesses seem to anticipate market shifts before they happen , while others are caught flat-footed? In today’s data-driven world, the difference lies in effective forecasting. Organizations that master the art and science of forecasting gain a competitive edge, with companies improving their planning accuracy by as much as 50%, dramatically affecting their net profit and strategic positioning. Whatever position you may be in, understanding forecasting’s core principles can help you transform how you approach decision-making and planning.
Understanding Forecasting: A Definitive Guide
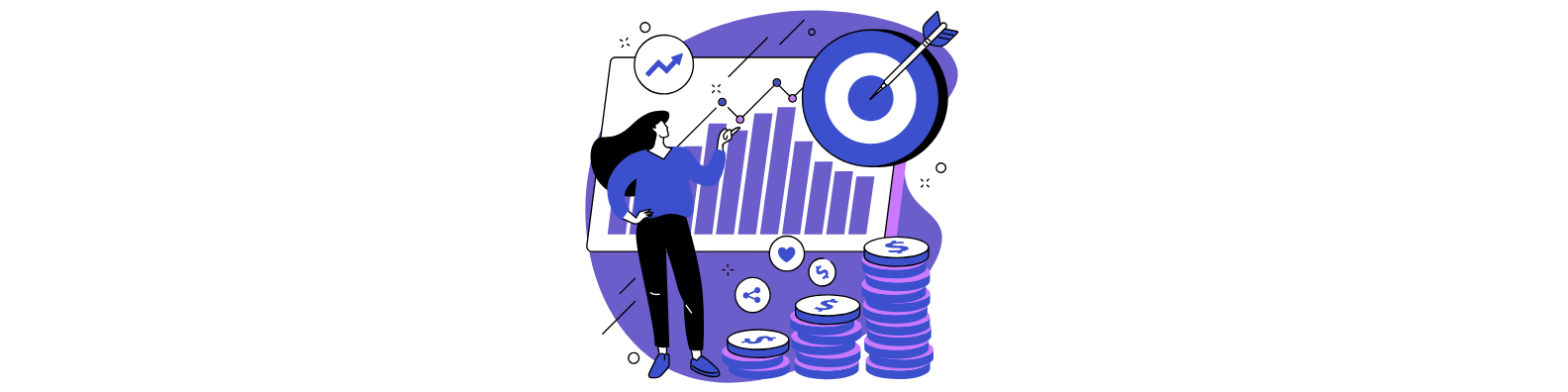
What is Forecasting?
Definition and Importance: Forecasting is the process of making informed predictions about future events based on past and present data, statistical analysis, and expert insights. Essentially, forecasting is a structured approach to anticipate what’s coming next. We need to recognize it as more than just an assumption, instead, let’s see it as a methodical technique that uses patterns and relationships in data to project future outcomes.
It helps organizations prepare for uncertainties by making it easier to allocate resources and develop responsive strategies.
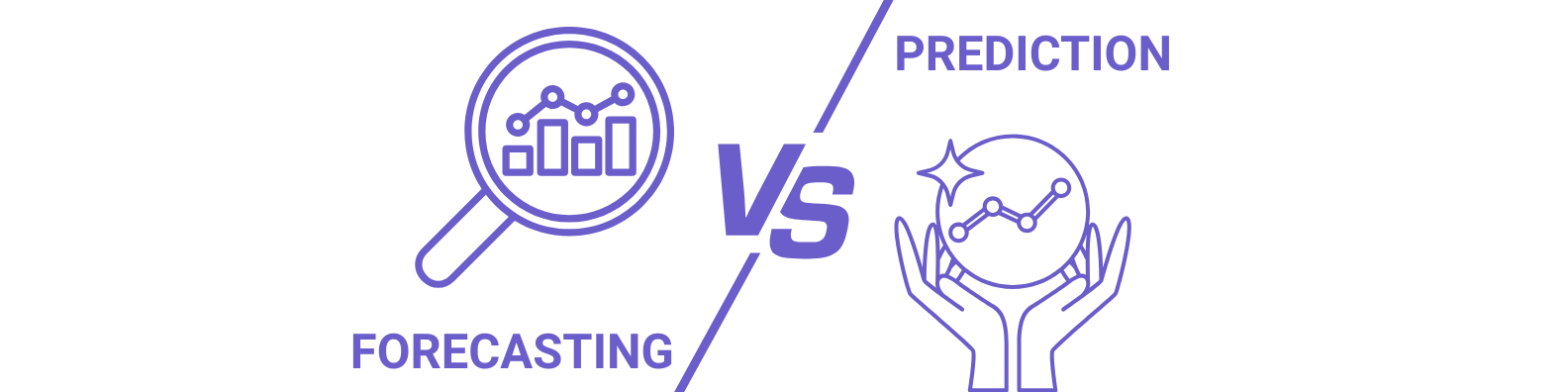
Forecasting vs. Prediction: Key Differences
While most people would often use these terms interchangeably, forecasting and prediction have distinct differences.
Forecasting involves a systematic process using historical data, statistical models, and defined methodologies to project future outcomes. Predictions, on the other hand, can be broader, less structured, and sometimes based more on intuition or opinion.
Furthermore, forecasting is more specific about the timeframe and conditions which offers a quantifiable outcome with confidence intervals and probabilities. While predictions provide a general outlook without the same level of statistical rigor.
You can think of it as forecasting saying, “Based on historical data and current trends, we expect our sales to increase by 12-15% next quarter”, while prediction be like, “I think our sales will go up soon.”
The Purpose of Forecasting

Application Across Industries
What do you think forecasting is used for? The applications span virtually every industry.
In cloud computing specifically, understanding usage patterns is important for accurate forecasting. This includes recognizing baseline usage and usage spikes, which can significantly impact costs. Different forecasting methods such as simple, trend-bases, and net new workload forecasting, have different strengths and are suitable for different scenarios depending on the business context.
Strategic Planning and Decision Making
In essence, forecasting exists to support better decisions. By providing insights into what might happen in the future, it gives decision-makers the data and information they need in order to develop strategies that account for various scenarios. This reduces uncertainty and helps organizations position themselves strategically.
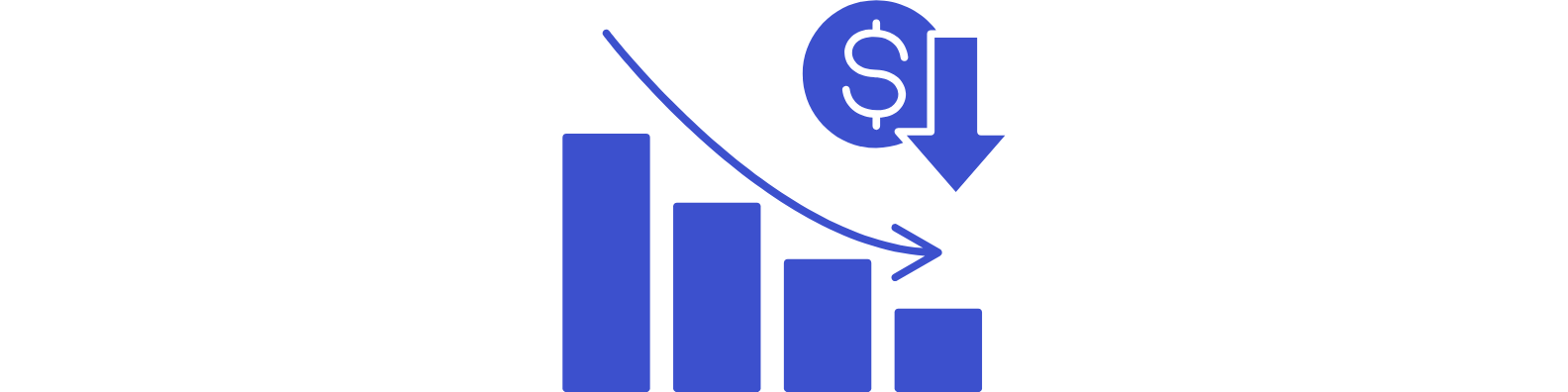
If we relate this to the FinOps framework, forecasting falls under the Inform phase, where we gather and analyze data to better understand current cloud spending before projecting future costs. This approach has been shown to improve efficiency, with some organizations reducing billing data processing needs up to 49% and enhancing cost allocation accuracy.
The goal isn't just to predict costs but to use those predictions to make smarter spending decisions.
Types of Forecasting Methods
Understanding forecasting techniques is crucial to effective forecasting. From traditional qualitative methods to sophisticated AI solutions, each approach offers unique advantages depending on your data, timeline, and objectives. Choosing the right approach is essential.
Let's explore various forecasting methods used by industry professionals.
Qualitative Forecasting Techniques

Qualitative forecasting methods are useful when there isn't much past data to rely on, like when launching a new product or entering a new market. In these cases, experts use their knowledge and experience to spot market trends. Market research also helps by collecting information about what customers like and what’s happening in the market. These methods give helpful insights when past data is limited.
One method, called the Delphi method, brings together a group of experts who give their opinions anonymously to avoid bias. The goal is to reach a shared prediction. Surveys are another tool, where customers are asked directly about what they plan to buy. Using both these methods, along with numbers and data, can lead to more accurate forecasts—especially in uncertain situations, like estimating cloud costs for a new project.
By combining expert opinions with data analysis, we get a clearer and more complete picture of future demand.
Emerging Methods in Forecasting
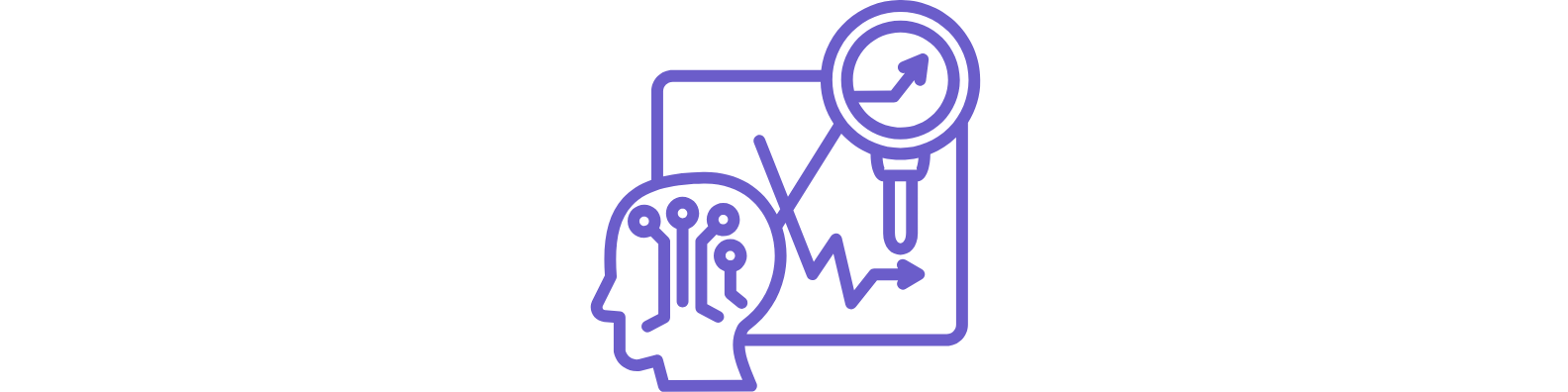
AI and machine learning are changing the way we forecast by quickly going through large amounts of data to find patterns and predict what might happen in the future. In managing cloud costs, AI tools can give real-time updates, find ways to save money, and help companies plan their future spending.
Using a mix of different forecasting methods can make predictions more accurate. This is especially helpful in cloud cost management, where AI can handle huge data sets to spot savings and forecast expenses more clearly.
Adaptive forecasting is a method where the system adjusts itself based on new information. This is useful in fast-changing markets or when dealing with new and innovative products.
The FinOps FOCUS framework is a good example of how combining forecasting methods can work well. It has helped improve efficiency and make cost tracking more accurate. This shows that modern forecasting methods can provide real business value, not just predictions.
The Process of Forecasting
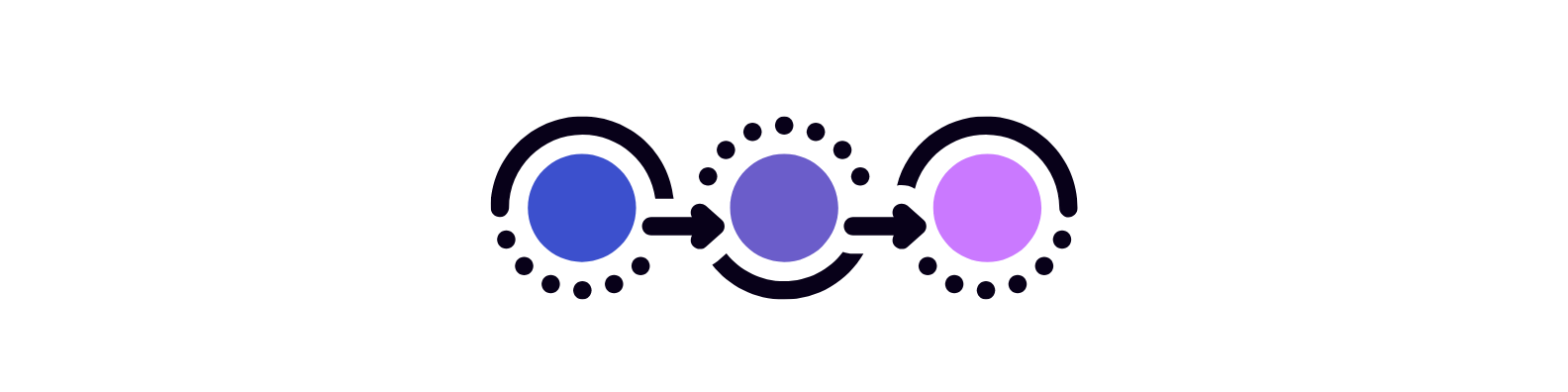
Creating accurate forecasts is not just about having the right tools. It is also about following the structured process that ensures reliability and relevance. Forecasting is a step-by-step process that turns data into useful predictions.
Let’s take a look at how good forecasting works from beginning to end.
- Identifying the Objective
The forecasting process always begins with a clear purpose. Before gathering data or selecting what model to use, you need to define what you are trying to predict and why.
Are you forecasting sales for planning?
Projecting cloud costs for budgeting?
Anticipating market trends for strategic decisions?
Each goal can help you decide how detailed and wide your forecast needs to be. In cloud cost management, knowing whether you're predicting steady, basic costs or changing, flexible workloads makes a big difference in how you plan your forecast.
- Collecting and Analyzing Data
Good data is the foundation of any accurate forecast. To make good predictions, you need to collect the right information that’s accurate and complete. This includes past data, current numbers, and anything else that might affect future outcomes. For cloud cost forecasting, that means looking at past usage, noticing both regular usage and sudden spikes, since both can affect how much things might cost later.
Analyzing the data is just as important. Before using it in forecasting tools, you should:
- Clean the data by removing mistakes or unusual numbers
- Look for patterns and trends over time
- Understand how different things affect each other
- Consider any special events or one-time changes
Doing this helps make sure your forecast is based on clear and useful information—not confusing or misleading data. The FinOps framework talks about this in its "Inform" phase, which focuses on collecting and understanding data before trying to predict future cloud costs.
- Choosing the Appropriate Model
Choosing the right forecasting method is an important part of the process. The best choice depends on several things, such as:
What kind of data do you have and how good it is?
How far into the future you want to predict?
How accurate your forecast needs to be?
What tools, time, and skills are available?
How complex are the connections between the things you're forecasting?
Sometimes, simple methods like moving averages work well when things are steady and predictable. For more complex situations with lots of changing factors, advanced tools like machine learning might be better.
There are different types of forecasting methods—like simple (naïve), trend-based, driver-based, or net new workload forecasting. Each one has its strengths and works best in different situations, depending on what your business needs.
Also, think about whether it’s more important to understand why the forecast says something (explanation), or if you just need accurate results (prediction). Some models help explain the reasons behind the numbers, while others are better at just giving solid estimates.
- Making and Communicating Predictions
Once you've chosen a forecasting model and used it with your data, the next step is to create the actual predictions. This means:
- Running the model with the right settings
- Checking if the results make sense
- Setting confidence levels (how sure you are about the results)
- Finding out what factors are affecting the forecast
But even a very accurate forecast doesn’t help much if people can’t understand it. That’s why it’s important to explain the results clearly in a way that makes sense for the business. Good communication includes showing the data visually (like in charts), pointing out the main findings, talking about what’s uncertain, and explaining what the forecast means for the business.
For example, when sharing a cloud cost forecast, you might show which workloads will likely raise costs, where there are chances to save money, or how future projects could affect spending. This turns the numbers into useful information that helps people make better decisions.
- Monitoring and Revising Forecasts
Forecasting doesn’t stop after making the first prediction. Good forecasting is an ongoing process that needs regular checking and improvement. By comparing what actually happened to what was predicted, you can:
- See how accurate your forecast was using standard measurements
- Spot any patterns of mistakes or bias
- Learn from the differences to make better forecasts in the future
- Update your models and settings if needed
It’s also important to set up regular feedback and create a culture where trying new ideas is encouraged. This means testing new ways of forecasting and celebrating when forecasts help the business make smart decisions.
Companies that are really good at forecasting treat it as something that keeps improving over time. As teams gain more experience, use better data, and try new technology, their forecasts become more advanced. Businesses that follow this kind of structured approach have seen big improvements—some even report up to 49% better accuracy.
Forecasting Accuracy and Challenges
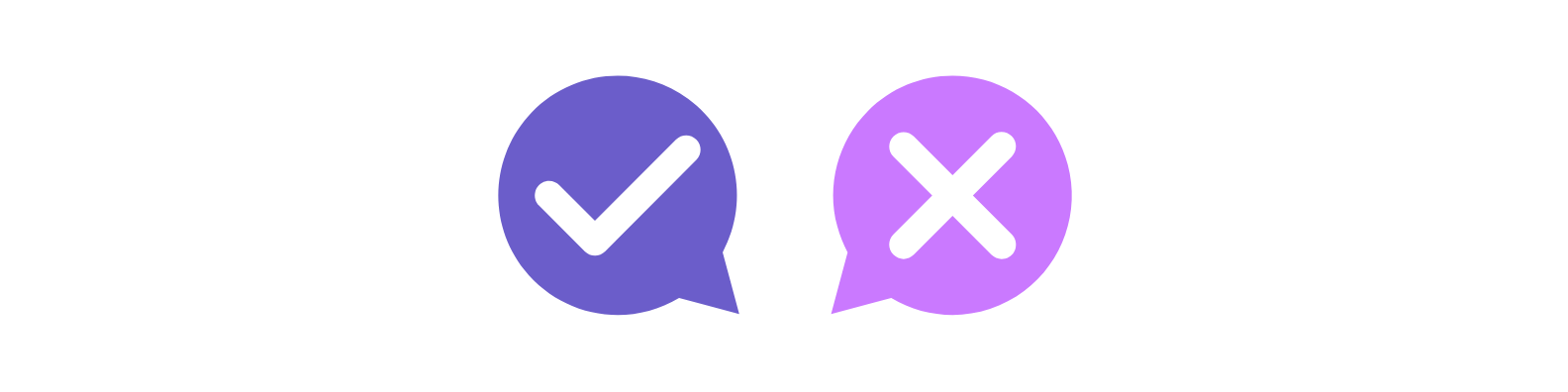
No matter how advanced your models are, forecasting always comes with some uncertainty. It’s important to know how to measure how well your forecast works and understand common mistakes that can affect accuracy. Let’s look at how to check forecasts and deal with common challenges.
Measuring Forecasting Accuracy
Error Metrics and Performance Indicators
To see how good a forecast is, we use certain numbers called error metrics. These help compare what was predicted with what actually happened:
- Mean Absolute Error: Shows the average size of mistakes. Lower is better.
- Mean Squared Error and Root Mean Squared Error: MSE is particularly useful when it comes to larger errors that are especially problematic. RMSE on the other hand, converts this back to the original unit, making it more interpretable while maintaining sensitivity to outliers.
- Mean Absolute Percentage Error: Shows errors as percentage. Helpful for comparing across different sizes, but not great when actual numbers are close to zero.
- Forecast Accuracy Percentage: Shows how close predictions were overall. 100% means a perfect forecast-easy for non-technical teams to understand.
Scaled Error Metrics
These help when comparing different data sets or time periods:
- MASE: Compare your forecast to a basic one. Less than 1 means your model is better.
- Theil’s U: Compares your forecast to a "no change" guess. Less than 1 means your forecast adds value.
In cloud cost forecasting, using these metrics helps companies check if their predictions are working and improve how they manage costs.
Common Challenges in Forecasting
Even the most sophisticated forecasting models can't overcome fundamental data problems. Here are some common data challenges that impact forecast performance:
- Incomplete historical data: The reliability of the forecast suffers when data is missing, inconsistently collected, or historically insufficient. This is particularly problematic when forecasting for new products or services where minimal historical data exists.
- Selection bias: The data you have access to may not represent the complete picture, leading to skewed forecasts. For instance, customer satisfaction surveys might predominantly capture very satisfied or very dissatisfied customers, missing the majority in between.
- Measurement errors: The accuracy of forecasts is directly dependent on the accuracy of the underlying data. In cloud cost management, incorrect tagging or miscategorized resources can significantly distort usage patterns, making accurate forecasting difficult.
- Changing relationships: Historical trends may not hold true as market conditions, customer behavior, and technology change. Understanding cloud usage patterns is crucial for accurate forecasting, including recognizing both baseline usage and usage spikes, which can significantly impact predicted costs.
To mitigate these challenges, organizations should invest in data quality processes, consider multiple data sources, and regularly audit their data collection methods to ensure they're capturing comprehensive, accurate information.
Forecast Smarter, Spend Wiser!
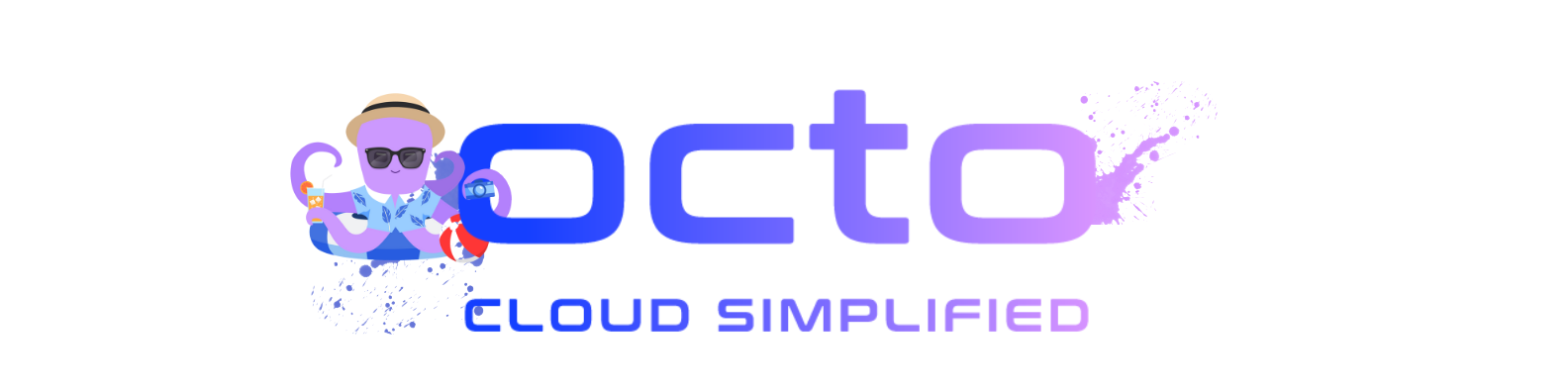
Forecasting isn’t just about guessing future costs—it’s about using your data to see what’s coming. Octo’s forecasting feature can help FinOps teams go from just reacting to budgets to planning ahead with confidence. It looks at past cloud usage, spots trends, and finds unusual cost spikes in real time, so you can predict future spending more accurately.
Whether you’re dealing with multiple cloud providers or quickly growing workloads, Octo adjusts to your needs and gives you easy-to-understand forecasts that match your business goals. It's designed to support the FinOps “Inform” phase by helping teams spot upcoming cost increases, reduce waste, and make smarter spending decisions—before surprise bills show up.
Stop flying blind with cloud costs. Let Octo bring forecasting into focus.
👉 See how Octo can transform your cloud cost strategy—book a demo today!