Imagine a world where your cloud costs are not only higher than expected but also harder to understand and control. Sadly, this is not just imagination but a reality many businesses face, especially when navigating the complexities of cloud computing. It’s like trying to follow a constantly shifting maze: you think you’ve found the way, only to discover another unexpected cost popping up somewhere else.
The truth is, this isn’t about occasional overspending; it’s about a fundamental shift in how we manage cloud costs and fully embrace FinOps.
Traditional budgeting methods and reactive cost‑cutting techniques often fall short and simply won’t do anymore. Sticking with the same old approach in the face of modern cost challenges is like trying to plug a leaky pipe with your finger—you might slow the drip for a moment, but you’ll never truly address the root cause. These constant firefighting efforts leave businesses frustrated and struggling to optimize their cloud spend.
How do we address these challenges?
The solution lies in anomaly detection, a rapidly growing field transforming data analysis and system protection. Projected to reach over $12 billion by 2029, this technology empowers businesses to proactively identify and address cost anomalies, turning potential budget overruns into opportunities for optimization and savings. Instead of reacting to unexpected expenses, teams can anticipate and prevent them—a shift from reactive to proactive cost management that’s crucial for successful FinOps. These algorithms act as silent guardians, detecting problems before they escalate into major issues.
Let’s explore the concept further.
Understanding Anomaly Detection
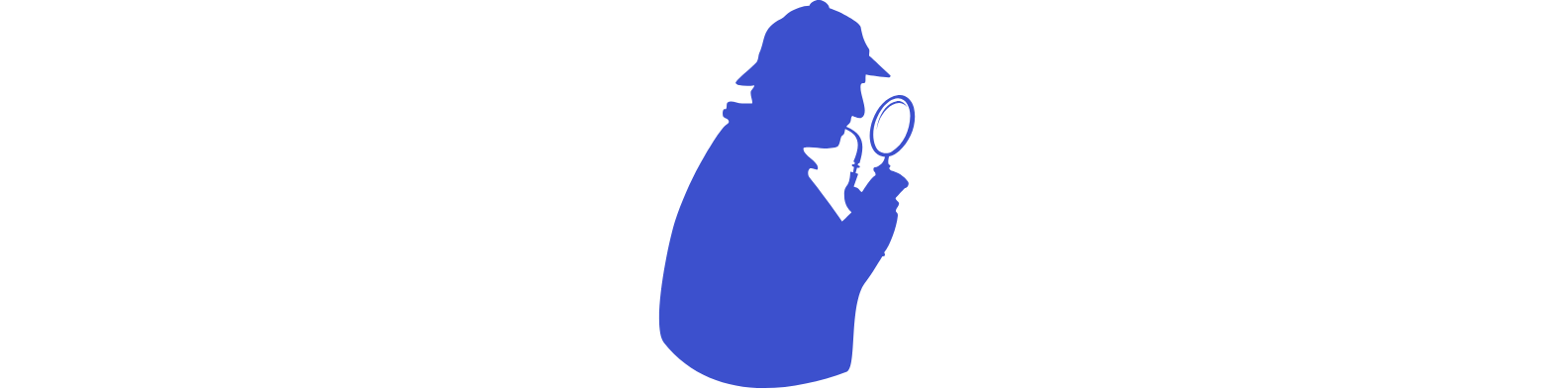
Definition and Key Concepts
Anomaly detection is exactly what it sounds like: finding things that don't fit the normal pattern.
But what does it really mean?
Simply put, anomaly detection is a process that identifies rare items, events, or observations that raise suspicions because they differ significantly from most of the data. Think of it as spotting that one odd sock in a big laundry pile; your brain naturally notices it because it doesn't match the pattern of the others. In data terms, anomaly detection works similarly, but with mathematical precision across massive datasets.
More technically, it's the process of identifying data points, observations, or events that significantly deviate from a dataset's normal behavior. These anomalies could signal problems, opportunities, or simply unusual activities worth investigating.
What makes anomaly detection so valuable is its versatility. It doesn't just tell you something unusual happened; it also helps you understand why and what to do about it. In today's data-driven world, that's incredibly valuable knowledge.
Historical Evolution of Anomaly Detection
Anomaly detection didn't begin with the AI algorithms we know today; it started with simple statistical methods. In the early days, analysts manually reviewed data, using basic statistical techniques to spot unusual patterns and outliers.
The early 1960s and 1970s saw the first formal statistical approaches for identifying anomalies. These methods relied heavily on assumptions about data distributions and were limited to smaller datasets that humans could reasonably analyze.
As computer power grew throughout the 1980s and 1990s, so did the sophistication of anomaly detection. Statistical methods evolved to handle larger datasets, and early machine learning techniques began to emerge. Rule-based systems became popular, especially in fraud detection and manufacturing quality control.
The real transformation came with the big data revolution of the 2000s. Businesses were suddenly collecting more data than ever before, making manual analysis impossible. This propelled anomaly detection into new territory—requiring automated, intelligent systems that could learn and adapt.
Today's anomaly detection uses sophisticated machine learning and deep learning approaches that can process billions of data points in real time. What started as simple statistical tests has evolved into complex neural networks capable of detecting subtle patterns invisible to the human eye.
The field continues to evolve rapidly, with each advancement making systems more intelligent at separating normal patterns from genuine anomalies—saving time, resources, and preventing significant damage across industries.
Importance and Benefits of Anomaly Detection

The importance of anomaly detection has grown exponentially as we’ve become more reliant on digital systems and data. As businesses navigate complex landscapes of threats and opportunities, spotting what’s out of place isn’t just helpful but also essential for survival and growth.
Enhancing Security and Compliance
Anomaly detection serves as an advanced early warning system. IT teams rely on it to spot suspicious activities that traditional rule-based systems might miss. When unusual login attempts or data access patterns occur, anomaly detection tools flag them before any damage is done.
Beyond external threats, anomaly detection strengthens internal security and compliance. It identifies unauthorized user access to resources or unusual movements of sensitive data. This capability is particularly valuable for industries facing strict regulatory requirements, where compliance failures can result in huge financial losses and reputational damage.
Furthermore, anomaly detection is a critical component in monitoring cloud environments. Sudden cost spikes could indicate not only budget issues but also potential security breaches, making it a valuable tool for both finance and IT teams.
Improving Operational Efficiency
Anomaly detection transforms how businesses maintain operational excellence. By identifying unusual patterns in equipment behavior or process flows, businesses can shift from reactive to proactive maintenance, fixing issues before they cause downtime.
In manufacturing environments, anomaly detection can improve operational efficiency by up to 25% through predictive maintenance and early detection of equipment issues. When a machine begins operating outside normal parameters, maintenance teams receive alerts before catastrophic failures occur.
For cloud-based operations, anomaly detection provides immediate insight into spending patterns, allowing for the quick identification of inefficiencies such as underutilized resources or misconfigured services. This real-time visibility helps prevent overspending and optimize resource allocation across complex infrastructure.
Most impressively, advanced anomaly detection systems learn over time. They understand seasonal trends and normal variations, reducing false alarms and ensuring that only genuine anomalies trigger alerts. This continuous improvement means teams focus on real issues, not noise.
Data-Driven Decision Making
Perhaps the most transformative benefit of anomaly detection is its ability to drive better business decisions. By highlighting deviations from expected patterns, it uncovers insights humans might never notice when looking at spreadsheets or dashboards.
Different teams use anomaly detection for varying purposes. Marketing teams use anomaly detection to identify unexpected changes in consumer behavior, allowing them to quickly adjust campaigns. Sales teams spot unusual buying patterns that might signal emerging market trends or competitive threats. Product teams identify unusual usage patterns that could point to either problems or opportunities for new features.
The technology acts as a force multiplier for analytics teams. Rather than sifting through mountains of data hoping to find something interesting, anomaly detection directs attention to the most relevant insights – the unusual data points that often hold important information.
As organizations accumulate more data, the importance of anomaly detection only grows. With proper implementation, it transforms overwhelming data volumes into clear signals that drive strategic advantage and operational excellence.
Types and Characteristics of Anomalies
Understanding the different types of anomalies in data is important for selecting the right detection approaches. Anomalies come in various forms, each requiring specific techniques to identify and address them effectively. Let’s explore the three main categories that form the foundation of anomaly detection.
Point Anomalies
These anomalies are the easiest to spot. Point anomalies represent the simplest and most common type of anomaly; they easily stand out, and you might immediately say, "That doesn't look right."
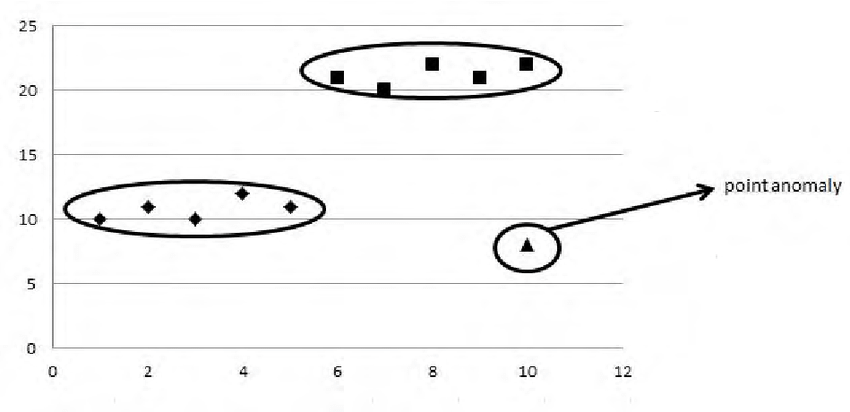
What makes point anomalies relatively straightforward to detect is their isolation. They don't require additional context to stand out. Statistical methods like standard deviation analysis or distance-based techniques can effectively flag these anomalies, making them the common type that basic detection systems look for.
Contextual Anomalies

These types of anomalies are much more sophisticated. They are data points that appear normal in isolation but become anomalous when viewed within a specific context, such as time, location, or user behavior patterns.
Consider it this way: certain data can be normal or suspicious depending on when or where it appears. For example, a $500 purchase in December might be perfectly normal, but the same purchase in April could raise eyebrows. Likewise, heavy network use at noon is expected, but at 3 AM, it might signal trouble. To detect these anomalies, the system needs to understand the context—such as time of day, season, and user habits—and adapt its expectations accordingly.
For businesses monitoring cloud costs, detecting these types of anomalies is particularly valuable. It can factor in seasonal trends and cyclical patterns, ensuring that only genuine anomalies trigger alerts, rather than expected variations like month-end processing or promotional activities.
Collective Anomalies
Collective anomalies represent the most sophisticated category of anomalies, where individual data points may seem normal, but together they form an unusual pattern. These anomalies are often the hardest to detect, yet they can uncover serious underlying issues.

Detecting collective anomalies requires advanced algorithms that can analyze the relationships and sequences between data points. Techniques such as graph-based methods, sequence analysis, and deep learning are particularly effective in these scenarios. However, defining what constitutes a "normal" collection of data points can be challenging and often requires domain expertise along with sophisticated modeling.
The ability to detect collective anomalies is crucial because they often reveal complex problems or opportunities that simpler anomaly types may miss. For instance, skilled fraudsters may avoid triggering point anomaly thresholds, but their collective behavior can still create detectable patterns.
Understanding the different types of anomalies—collective, point, and contextual—forms the basis for effective detection systems. Robust platforms and systems typically incorporate techniques for all three types, providing layered defenses against unexpected events. As data complexity increases, the ability to identify and categorize these anomalies becomes vital for businesses aiming to protect their assets and optimize operations.
Techniques and Methods for Anomaly Detection

The field of anomaly detection has evolved dramatically over the years, with techniques ranging from simple statistical tests to sophisticated neural networks. Choosing the right anomaly detection algorithms for your specific use case is crucial for success. Let’s explore the major approaches that power today’s detection systems.
Statistical Methods
Statistical methods represent the oldest and most established anomaly detection methods. These approaches rely on mathematical principles to identify data points that deviate significantly from expected distributions.
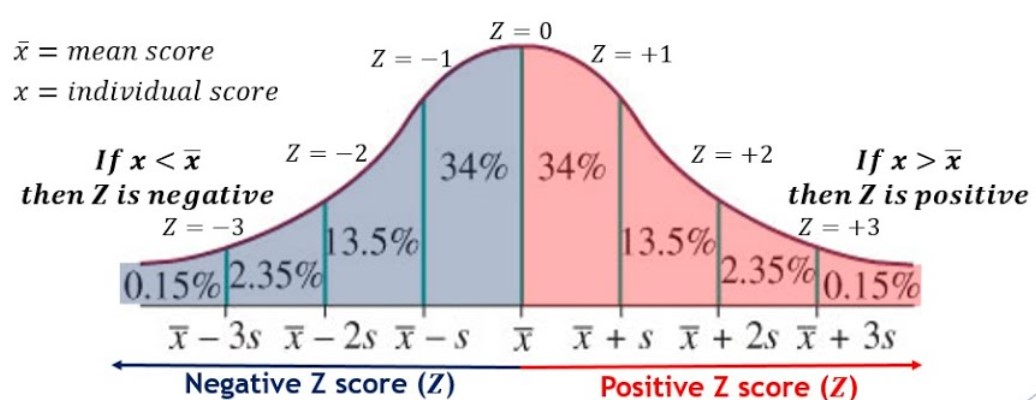
- Z-Score Analysis: This is a straightforward and effective technique that assesses how far a data point is from the average by measuring its number of standard deviations from the mean. For example, if a value exceeds a certain threshold—such as a Z-score above 3 in financial transaction monitoring—it may trigger an investigation for potential fraud.

- Interquartile Range (IQR): It is a useful method for identifying anomalies, particularly in data with skewed distributions. It focuses on the middle 50% of the data to determine normal ranges, allowing it to detect anomalies without assuming a specific distribution.
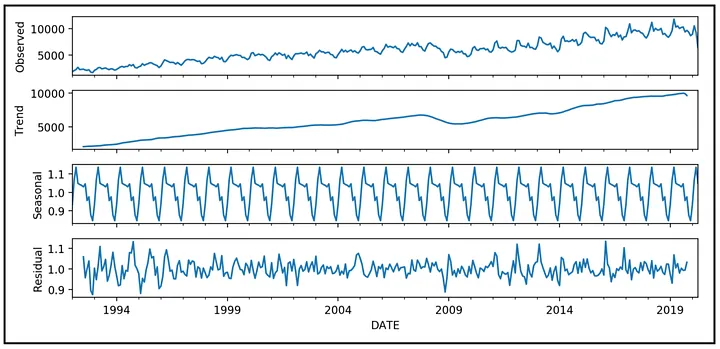
- Time Series Decomposition: This technique is particularly valuable for data with seasonal or cyclical patterns. It breaks down the data into trend, seasonal, and residual components, allowing analysts to identify anomalies within the appropriate context.
Machine Learning Approaches
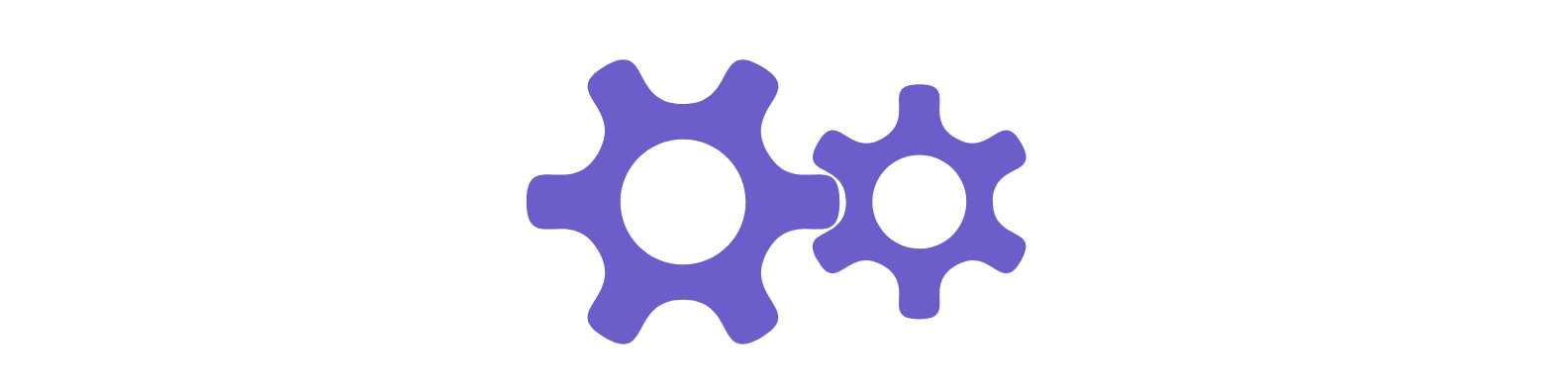
Machine learning has revolutionized anomaly detection by enabling systems to learn complex patterns autonomously. It uses three main approaches: supervised, unsupervised, and semi-supervised.
- Supervised Learning: Requires labeled datasets with examples of both normal and anomalous instances, however, the challenge with this approach lies in obtaining labeled anomaly examples. In many real-world scenarios, anomalies are rare or constantly evolving, making comprehensive labeled datasets difficult to create. But, if a good training data exists, supervised methods often deliver the highest accuracy and lowers false positive rates.
- Unsupervised Learning: The type of approach addresses the problem in the previous example. While supervised learning requires labeled data sets, unsupervised learning identifies anomalies with requiring examples. This technique analyzes inherent data structures to determine what’s normal and what’s not. It is particularly useful when dealing with unknown anomalies or evolving environments where defining ‘normal’ is challenging, which is particularly useful in cybersecurity where threats constantly evolve to evade detection.
- Semi-Supervised Learning (Reinforcement): It strikes a balance between supervised and unsupervised learning approaches. Using a small amount of labeled data (typically just normal examples) alongside a large amount of unlabeled data improves detection accuracy. This semi-supervised approach offers a practical advantage in many real-world scenarios where collecting comprehensive anomaly examples is impractical, but some labeled normal data is readily available.
Deep Learning Architectures
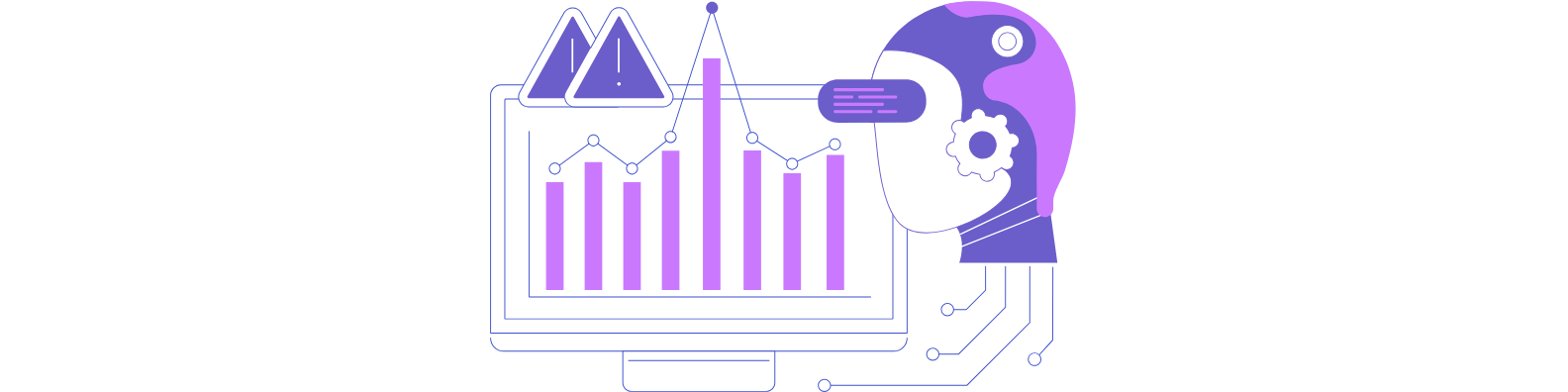
Deep learning has significantly advanced anomaly detection, especially for complex data like images, videos, and text. Its sophisticated algorithms can find hidden patterns that simpler methods miss, delivering excellent results. However, deep learning requires more computing power and its results can be harder to understand. Many businesses use it with simpler methods, creating layered detection systems that balance performance and resource use.
Common Implementation Challenges
Despite impressive capabilities, organizations implementing anomaly detection face significant hurdles. Understanding these challenges is crucial for successful deployment:
- Data Quality and Quantity: This is the most fundamental challenge. Effective anomaly detection needs large amounts of clean, relevant data to establish reliable baselines. Organizations often find their data collection is inadequate only after starting implementation. Poor data quality leads to inaccurate results and ineffective systems.
- False Positives: Many systems generate too many alerts for non-issues, leading to alert fatigue and missed critical events. Properly tuning sensitivity requires ongoing adjustments and expertise.
- Computational Requirements: Processing massive datasets requires significant computing power, making real-time detection in large environments difficult for many organizations without cloud solutions.
- Integration Challenges: Implementing anomaly detection within existing workflows can be difficult. Poor planning can cause even excellent systems to fail if they don't fit with operational processes or existing technology.
- Dynamic Environments: Constantly changing environments, such as cloud infrastructures, present challenges. Defining normal is difficult when the baseline itself is constantly shifting.
Building and Optimizing Anomaly Detection Systems
Creating effective anomaly detection requires thoughtful planning and ongoing optimization. Here are the key considerations for building robust systems:
- Start with clear objectives. Define what constitutes an anomaly in your specific context and prioritize detection based on business impact. Not all anomalies deserve equal attention.
- Invest in data preparation. Clean, normalized data dramatically improves detection accuracy. Establish processes for data collection and preprocessing before implementation.
- Layer multiple detection methods. Combine statistical, machine learning, and rule-based approaches to create defense in depth. Different techniques catch different types of anomalies.
- Implement feedback loops. Systems should learn from false positives and missed anomalies. Create processes for analysts to provide feedback that improves models over time.
- Consider human factors. Design interfaces that present anomalies with appropriate context, supporting human decision-making rather than overwhelming analysts.
- Plan for scale. As data volumes grow, ensure your architecture can scale accordingly, whether through cloud resources or distributed processing.
- Maintain domain expertise involvement. The most successful implementations maintain close collaboration between data scientists and domain experts who understand normal operations.
Step-Up Your Anomaly Detection with Octo!
Businesses that adopt these best practices can build anomaly detection systems that deliver actionable insights while minimizing false alarms and inefficiencies. The most effective implementations treat anomaly detection as an iterative process—not a static solution—continuously adapting to new patterns through tools like Octo. By analyzing data against historical baselines, Octo identifies anomalous data with precision, enabling businesses to refine detection logic and stay ahead of emerging risks.

With threats getting more complex and data growing rapidly, strong anomaly detection is no longer optional—it's essential. Companies using smart detection systems get more than just better security; they gain valuable insights to make better decisions, improve processes, and stay ahead of the competition. Octo helps businesses turn anomalies into opportunities for innovation and growth, turning uncertainty into a path to success.
Ready to step up your anomaly detection? Book a demo today to learn more.